Method Overview
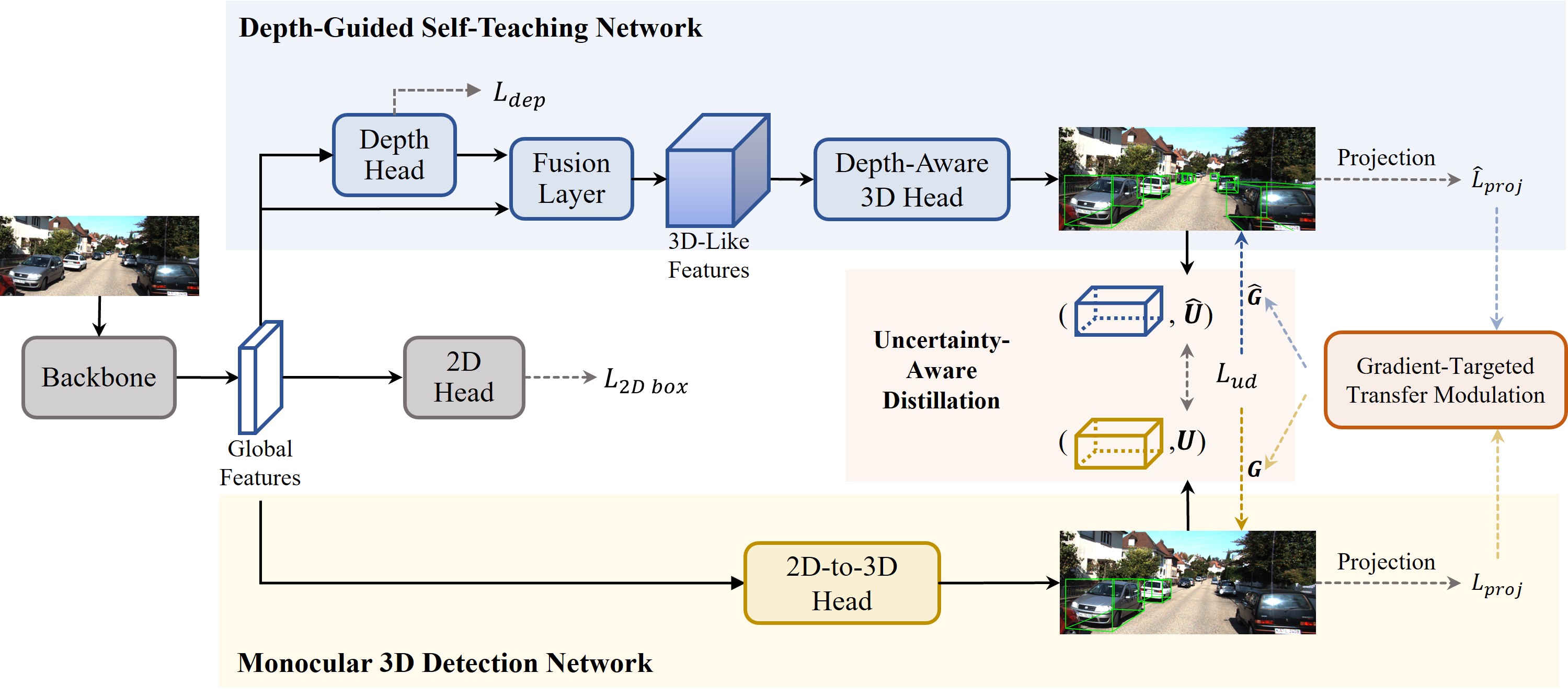
The framework of the proposed self-knowledge distillation network. The framework consists of a depth-guided self-teaching network and a monocular 3D detection network. The depth-guided self-teaching network acquires comprehensive 3D localization knowledge by leveraging depth information and transfers its learned expertise to the monocular 3D detection network via soft label distillation to enhance its performance. We design an uncertainty-aware distillation loss and a gradient-targeted transfer modulation strategy to facilitate the knowledge transfer between the two networks effectively. During inference, the monocular 3D detection network extracts intrinsic depth information from single-view images independently with little computational overhead.